Stochastic Programming and Scenario Generation: Decision Modelling Simulation and Information systems Perspective
Stochastic Programming brings together models of optimum resource allocation and models of randomness and thereby creates a robust decision making framework. The models of randomness with their finite, discrete realisations are known as Scenario Generators. In this thesis we consider alternative approaches to Scenario Generation (SG) in a generic form which can be used to formulate (a) two stage (static) and (b) multistage dynamic SP models. We also investigate the modelling structure and software issues of integrating a scenario generator with an optimisation model to construct Stochastic Programming recourse problems. We consider how the Expected Value (EV) and Stochastic Programming (SP) decision model results can be evaluated within a descriptive modelling framework of simulation. Illustrative examples and computational results are given in support of our investigation. We set out a blueprint for a SG model library; its open architecture and how it is used in decision models and in simulation.
The major contribution of this research reported in this thesis is the development of a two phase modelling paradigm which connects forward looking (ex ante) decision making with wait and see (ex post) results analysis. The research also connects these modelling paradigms to the analytic information systems, which are used by decision makers of diverse organisations. This latter aspect, which integrates information engineering with analytics decision models taking into consideration uncertainty and risk, is a contribution to knowledge. The scenario generation, the simulation extension to SAMPL and the design of the SG library encapsulate a number of novel features and are also a contribution to knowledge.
CONSULTA INTEGRALMENTE QUESTA TESI
La consultazione è esclusivamente in formato digitale .PDF
Acquista
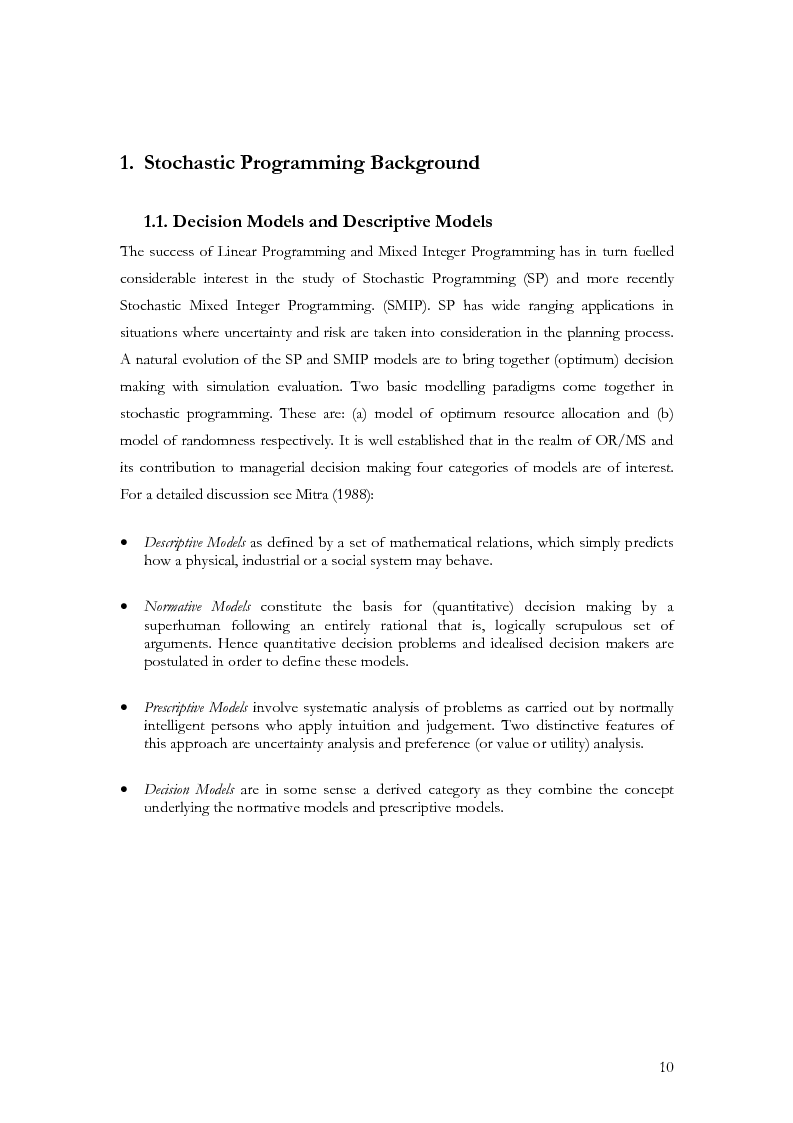
CONSULTA INTEGRALMENTE QUESTA TESI
La consultazione è esclusivamente in formato digitale .PDF
Acquista
L'unico servizio antiplagio competitivo nel prezzo che garantisce l'aiuto della nostra redazione nel controllo dei risultati.
Analisi sicura e anonima al 100%!
Ottieni un Certificato Antiplagio dopo la valutazione.
Informazioni tesi
Autore: | Nico Di Domenica |
Tipo: | Tesi di Dottorato |
Dottorato in | Degree of Doctor of Philosophy |
Anno: | 2005 |
Docente/Relatore: | Gautam Mitra |
Correlatore: | CormacLucas |
Istituito da: | Brunel University |
Dipartimento: | Mathematics |
Lingua: | Inglese |
Num. pagine: | 140 |
Forse potrebbe interessarti la tesi:
The Simulation Approach for the Study of Electoral Systems
FAQ
Come consultare una tesi
Il pagamento può essere effettuato tramite carta di credito/carta prepagata, PayPal, bonifico bancario.
Confermato il pagamento si potrà consultare i file esclusivamente in formato .PDF accedendo alla propria Home Personale. Si potrà quindi procedere a salvare o stampare il file.
Maggiori informazioni
Perché consultare una tesi?
- perché affronta un singolo argomento in modo sintetico e specifico come altri testi non fanno;
- perché è un lavoro originale che si basa su una ricerca bibliografica accurata;
- perché, a differenza di altri materiali che puoi reperire online, una tesi di laurea è stata verificata da un docente universitario e dalla commissione in sede d'esame. La nostra redazione inoltre controlla prima della pubblicazione la completezza dei materiali e, dal 2009, anche l'originalità della tesi attraverso il software antiplagio Compilatio.net.
Clausole di consultazione
- L'utilizzo della consultazione integrale della tesi da parte dell'Utente che ne acquista il diritto è da considerarsi esclusivamente privato.
- Nel caso in cui l’utente che consulta la tesi volesse citarne alcune parti, dovrà inserire correttamente la fonte, come si cita un qualsiasi altro testo di riferimento bibliografico.
- L'Utente è l'unico ed esclusivo responsabile del materiale di cui acquista il diritto alla consultazione. Si impegna a non divulgare a mezzo stampa, editoria in genere, televisione, radio, Internet e/o qualsiasi altro mezzo divulgativo esistente o che venisse inventato, il contenuto della tesi che consulta o stralci della medesima. Verrà perseguito legalmente nel caso di riproduzione totale e/o parziale su qualsiasi mezzo e/o su qualsiasi supporto, nel caso di divulgazione nonché nel caso di ricavo economico derivante dallo sfruttamento del diritto acquisito.
Vuoi tradurre questa tesi?
Per raggiungerlo, è fondamentale superare la barriera rappresentata dalla lingua. Ecco perché cerchiamo persone disponibili ad effettuare la traduzione delle tesi pubblicate nel nostro sito.
Per tradurre questa tesi clicca qui »
Scopri come funziona »
DUBBI? Contattaci
Contatta la redazione a
[email protected]
Parole chiave
Tesi correlate
Non hai trovato quello che cercavi?
Abbiamo più di 45.000 Tesi di Laurea: cerca nel nostro database
Oppure consulta la sezione dedicata ad appunti universitari selezionati e pubblicati dalla nostra redazione
Ottimizza la tua ricerca:
- individua con precisione le parole chiave specifiche della tua ricerca
- elimina i termini non significativi (aggettivi, articoli, avverbi...)
- se non hai risultati amplia la ricerca con termini via via più generici (ad esempio da "anziano oncologico" a "paziente oncologico")
- utilizza la ricerca avanzata
- utilizza gli operatori booleani (and, or, "")
Idee per la tesi?
Scopri le migliori tesi scelte da noi sugli argomenti recenti
Come si scrive una tesi di laurea?
A quale cattedra chiedere la tesi? Quale sarà il docente più disponibile? Quale l'argomento più interessante per me? ...e quale quello più interessante per il mondo del lavoro?
Scarica gratuitamente la nostra guida "Come si scrive una tesi di laurea" e iscriviti alla newsletter per ricevere consigli e materiale utile.
La tesi l'ho già scritta,
ora cosa ne faccio?
La tua tesi ti ha aiutato ad ottenere quel sudato titolo di studio, ma può darti molto di più: ti differenzia dai tuoi colleghi universitari, mostra i tuoi interessi ed è un lavoro di ricerca unico, che può essere utile anche ad altri.
Il nostro consiglio è di non sprecare tutto questo lavoro:
È ora di pubblicare la tesi