An Exploration of the Introduction of Warping to the Eigenfaces
The aim of this work is to provide a robust and reliable model of the face by exploring the benefits of the introduction of warping as a pre-processing technique to improve the result of the eigen faces statistical analysis. We prove the advantages of such an approach both from a theoretical and experimental perspective.
This model is based on all the previous works listed but it has a different approach to the problem of modelling the face space : on the theoretical side we try to eradicate the sources of noise and variance before performing the face analysis rather than trying to minimise them during the process. From the practical point of view
we introduce a complete modularity of the processes therefore we reduce the risk of side effects and correlation that might reduce the reliability of the results. Moreover we can add other pre-processing or post processing techniques to improve the results without affecting what we have already achieved.
The key issue is the eigen face as everything we studied and all this work has been done to improve the performance of this technique but we prove that this technique is a valid and reliable approach to the problem.
We approached both the shape variance and the lighting variance from a slightly different perspective to create a technique that is widely usable and can be adapted to any particular set of data by changing one of the two algorithms involved leaving the other one unaffected.
We have applied the "Douglas Smythe" ( 1990 ) algorithm to warp a training set of 21 colour images as a pre-processing technique of the eigen-faces analysis.
This approach is motivated by the intrinsic nature of the eigen-faces where all the important features of the faces are supposed to be aligned. In real life this is quite unlikely to happen and the result is blurred. But, if we apply a warping technique to all the images of our training set and we warp them to any arbitrary image, within this set, before performing the eigen faces; the images will have exactly the same features in the same spatial coordinates. This should dramatically improve the performance of the eigen technique.
Moreover we have a set of landmarks and offsets that can be used to perform a statistical analysis to describe how these point vary in our training set that describes the spatial variance of the main features of the faces in the training set we use. The applications are various: we can use this program as a platform to achieve image recognition or to create a large number of new faces from the training set or we can use it to add and remove facial expressions, locate a face in an image and to achieve a more "natural" animation
CONSULTA INTEGRALMENTE QUESTA TESI
La consultazione è esclusivamente in formato digitale .PDF
Acquista
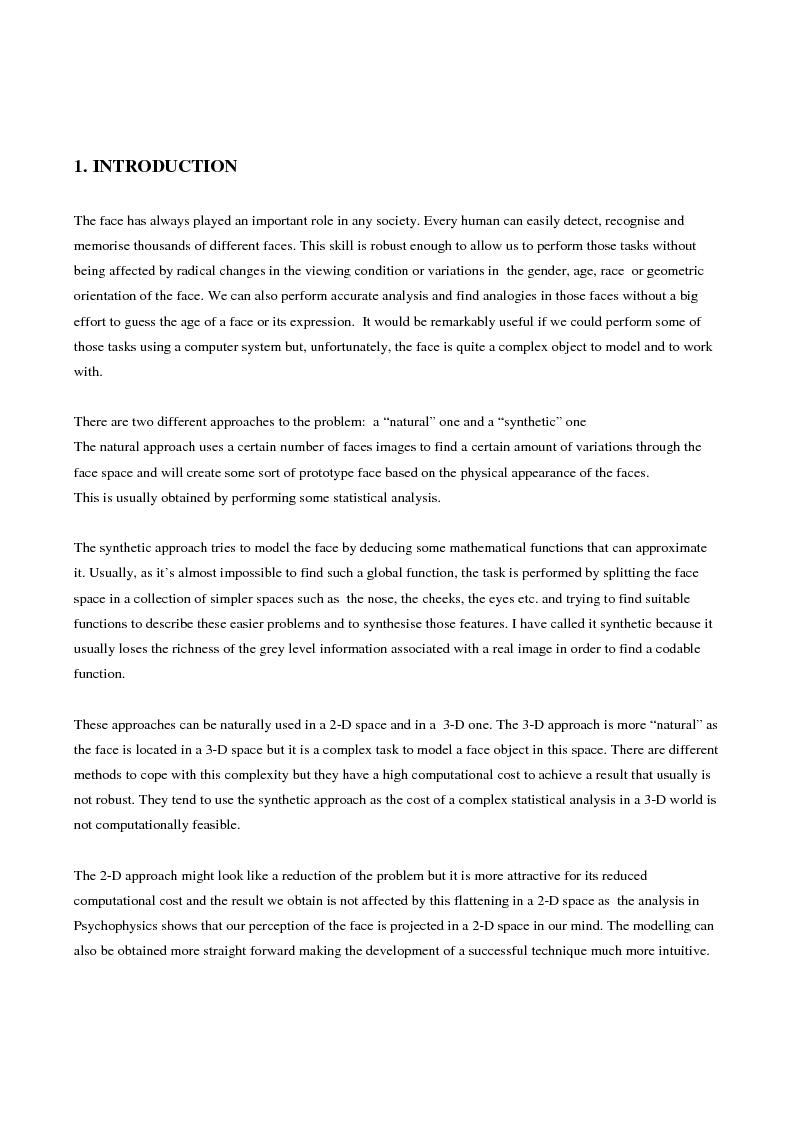
CONSULTA INTEGRALMENTE QUESTA TESI
La consultazione è esclusivamente in formato digitale .PDF
Acquista
L'unico servizio antiplagio competitivo nel prezzo che garantisce l'aiuto della nostra redazione nel controllo dei risultati.
Analisi sicura e anonima al 100%!
Ottieni un Certificato Antiplagio dopo la valutazione.
Informazioni tesi
Autore: | Luca Sorbello |
Tipo: | Tesi di Laurea |
Anno: | 1998-99 |
Università: | University of Leeds |
Facoltà: | Scienze Matematiche, Fisiche e Naturali |
Corso: | Scienze dell'Informazione |
Relatore: | David Hogg |
Lingua: | Inglese |
Num. pagine: | 54 |
Forse potrebbe interessarti la tesi:
Computer Vision - Principi di Head Detection e di Hand Tracking
FAQ
Come consultare una tesi
Il pagamento può essere effettuato tramite carta di credito/carta prepagata, PayPal, bonifico bancario.
Confermato il pagamento si potrà consultare i file esclusivamente in formato .PDF accedendo alla propria Home Personale. Si potrà quindi procedere a salvare o stampare il file.
Maggiori informazioni
Perché consultare una tesi?
- perché affronta un singolo argomento in modo sintetico e specifico come altri testi non fanno;
- perché è un lavoro originale che si basa su una ricerca bibliografica accurata;
- perché, a differenza di altri materiali che puoi reperire online, una tesi di laurea è stata verificata da un docente universitario e dalla commissione in sede d'esame. La nostra redazione inoltre controlla prima della pubblicazione la completezza dei materiali e, dal 2009, anche l'originalità della tesi attraverso il software antiplagio Compilatio.net.
Clausole di consultazione
- L'utilizzo della consultazione integrale della tesi da parte dell'Utente che ne acquista il diritto è da considerarsi esclusivamente privato.
- Nel caso in cui l’utente che consulta la tesi volesse citarne alcune parti, dovrà inserire correttamente la fonte, come si cita un qualsiasi altro testo di riferimento bibliografico.
- L'Utente è l'unico ed esclusivo responsabile del materiale di cui acquista il diritto alla consultazione. Si impegna a non divulgare a mezzo stampa, editoria in genere, televisione, radio, Internet e/o qualsiasi altro mezzo divulgativo esistente o che venisse inventato, il contenuto della tesi che consulta o stralci della medesima. Verrà perseguito legalmente nel caso di riproduzione totale e/o parziale su qualsiasi mezzo e/o su qualsiasi supporto, nel caso di divulgazione nonché nel caso di ricavo economico derivante dallo sfruttamento del diritto acquisito.
Vuoi tradurre questa tesi?
Per raggiungerlo, è fondamentale superare la barriera rappresentata dalla lingua. Ecco perché cerchiamo persone disponibili ad effettuare la traduzione delle tesi pubblicate nel nostro sito.
Scopri come funziona »
DUBBI? Contattaci
Contatta la redazione a
[email protected]
Parole chiave
Tesi correlate
Non hai trovato quello che cercavi?
Abbiamo più di 45.000 Tesi di Laurea: cerca nel nostro database
Oppure consulta la sezione dedicata ad appunti universitari selezionati e pubblicati dalla nostra redazione
Ottimizza la tua ricerca:
- individua con precisione le parole chiave specifiche della tua ricerca
- elimina i termini non significativi (aggettivi, articoli, avverbi...)
- se non hai risultati amplia la ricerca con termini via via più generici (ad esempio da "anziano oncologico" a "paziente oncologico")
- utilizza la ricerca avanzata
- utilizza gli operatori booleani (and, or, "")
Idee per la tesi?
Scopri le migliori tesi scelte da noi sugli argomenti recenti
Come si scrive una tesi di laurea?
A quale cattedra chiedere la tesi? Quale sarà il docente più disponibile? Quale l'argomento più interessante per me? ...e quale quello più interessante per il mondo del lavoro?
Scarica gratuitamente la nostra guida "Come si scrive una tesi di laurea" e iscriviti alla newsletter per ricevere consigli e materiale utile.
La tesi l'ho già scritta,
ora cosa ne faccio?
La tua tesi ti ha aiutato ad ottenere quel sudato titolo di studio, ma può darti molto di più: ti differenzia dai tuoi colleghi universitari, mostra i tuoi interessi ed è un lavoro di ricerca unico, che può essere utile anche ad altri.
Il nostro consiglio è di non sprecare tutto questo lavoro:
È ora di pubblicare la tesi