Applying multi-objective optimization to a ventilation duct for an automotive vehicle
Optimization is the task of finding solutions to a problem until no better ones can be found. The search process is aimed at finding the extremes of one or more objectives. Often the objectives are restricted by one or more constraints. In multi-objective optimization the number of objective functions is more than one, making the task of finding the optimum a difficult one especially if objectives are conflicting.
In this study we have applied optimization techniques to an automotive ventilation duct. The problem originates from the real-world demand of determining an optimal duct shape during the early stages of car development. Traditionally, optimization is driven by engineering decisions and applied late in the development process. We have followed the different approach of employing automatic process-based optimization and considering a simplified geometry suitable for the early phases of a project. The ultimate goal was to provide feasibility and design indications.
During the study we have developed a simplified geometrical model of right ventilation duct. This was used as the starting point for building the optimization process in terms of design variables. The objectives were set to minimize the pressure losses and the noise levels in the duct. Constraints were limited to the project’s geometrical bounds and extremes.
However, the initial fluid dynamic objectives have been changed throughout the study to geometrical only principles in order to avoid the computational expense of CFD simulations. To correlate with the initial objectives a CFD analysis was performed on the results at the end of the process. The new objectives were set to achieve maximal duct uniformity and minimal surface curvature.
To optimize the duct geometry a multi-level approach was chosen. The significant number of parameters involved and the discontinuities in the search space demanded for an incremental approach rather than a direct optimization. We successfully optimized the geometry by performing an initial Design Of Experiments to seek for feasible solutions followed by three optimization runs with evolutionary algorithms to achieve uniformity and minimal curvature.
Results have proven how the geometrical principles can replace successfully the fluid dynamic objectives and still obtain optimal solutions. Pressure losses and noise levels decreased constantly from the initial geometrical setup onwards to final results.
CONSULTA INTEGRALMENTE QUESTA TESI
La consultazione è esclusivamente in formato digitale .PDF
Acquista
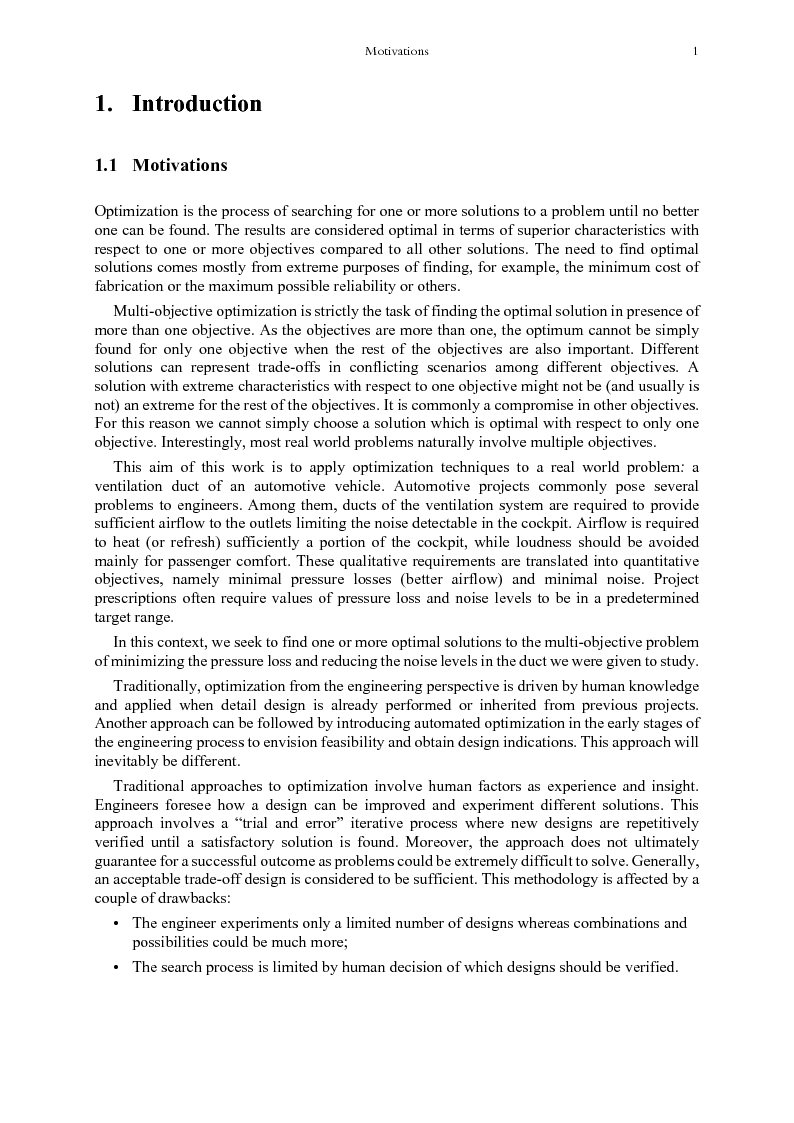
CONSULTA INTEGRALMENTE QUESTA TESI
La consultazione è esclusivamente in formato digitale .PDF
Acquista
Informazioni tesi
Autore: | Eugenio Giulio Rizzi |
Tipo: | Laurea I ciclo (triennale) |
Anno: | 2010-11 |
Università: | Politecnico di Torino |
Facoltà: | Ingegneria |
Corso: | Ingegneria Aerospaziale |
Lingua: | Inglese |
Num. pagine: | 99 |
Forse potrebbe interessarti la tesi:
Sviluppo ed ottimizzazione dello sterzo di un veicolo da competizione
FAQ
Come consultare una tesi
Il pagamento può essere effettuato tramite carta di credito/carta prepagata, PayPal, bonifico bancario.
Confermato il pagamento si potrà consultare i file esclusivamente in formato .PDF accedendo alla propria Home Personale. Si potrà quindi procedere a salvare o stampare il file.
Maggiori informazioni
Perché consultare una tesi?
- perché affronta un singolo argomento in modo sintetico e specifico come altri testi non fanno;
- perché è un lavoro originale che si basa su una ricerca bibliografica accurata;
- perché, a differenza di altri materiali che puoi reperire online, una tesi di laurea è stata verificata da un docente universitario e dalla commissione in sede d'esame. La nostra redazione inoltre controlla prima della pubblicazione la completezza dei materiali e, dal 2009, anche l'originalità della tesi attraverso il software antiplagio Compilatio.net.
Clausole di consultazione
- L'utilizzo della consultazione integrale della tesi da parte dell'Utente che ne acquista il diritto è da considerarsi esclusivamente privato.
- Nel caso in cui l’utente che consulta la tesi volesse citarne alcune parti, dovrà inserire correttamente la fonte, come si cita un qualsiasi altro testo di riferimento bibliografico.
- L'Utente è l'unico ed esclusivo responsabile del materiale di cui acquista il diritto alla consultazione. Si impegna a non divulgare a mezzo stampa, editoria in genere, televisione, radio, Internet e/o qualsiasi altro mezzo divulgativo esistente o che venisse inventato, il contenuto della tesi che consulta o stralci della medesima. Verrà perseguito legalmente nel caso di riproduzione totale e/o parziale su qualsiasi mezzo e/o su qualsiasi supporto, nel caso di divulgazione nonché nel caso di ricavo economico derivante dallo sfruttamento del diritto acquisito.
Vuoi tradurre questa tesi?
Per raggiungerlo, è fondamentale superare la barriera rappresentata dalla lingua. Ecco perché cerchiamo persone disponibili ad effettuare la traduzione delle tesi pubblicate nel nostro sito.
Per tradurre questa tesi clicca qui »
Scopri come funziona »
DUBBI? Contattaci
Contatta la redazione a
[email protected]
Parole chiave
Tesi correlate
Non hai trovato quello che cercavi?
Abbiamo più di 45.000 Tesi di Laurea: cerca nel nostro database
Oppure consulta la sezione dedicata ad appunti universitari selezionati e pubblicati dalla nostra redazione
Ottimizza la tua ricerca:
- individua con precisione le parole chiave specifiche della tua ricerca
- elimina i termini non significativi (aggettivi, articoli, avverbi...)
- se non hai risultati amplia la ricerca con termini via via più generici (ad esempio da "anziano oncologico" a "paziente oncologico")
- utilizza la ricerca avanzata
- utilizza gli operatori booleani (and, or, "")
Idee per la tesi?
Scopri le migliori tesi scelte da noi sugli argomenti recenti
Come si scrive una tesi di laurea?
A quale cattedra chiedere la tesi? Quale sarà il docente più disponibile? Quale l'argomento più interessante per me? ...e quale quello più interessante per il mondo del lavoro?
Scarica gratuitamente la nostra guida "Come si scrive una tesi di laurea" e iscriviti alla newsletter per ricevere consigli e materiale utile.
La tesi l'ho già scritta,
ora cosa ne faccio?
La tua tesi ti ha aiutato ad ottenere quel sudato titolo di studio, ma può darti molto di più: ti differenzia dai tuoi colleghi universitari, mostra i tuoi interessi ed è un lavoro di ricerca unico, che può essere utile anche ad altri.
Il nostro consiglio è di non sprecare tutto questo lavoro:
È ora di pubblicare la tesi