Mixture models for count data: the ZIP model
The thesis, done under the Generalized Linear Models (GLM), is divided into theoretical development before then applied to the problem of excess zeros within a dataset insurance, kindly offered by ANIA. This dataset was then modeled with a template ZIP: "Zero Inflated Poisson." Before applying carried out with the statistical software R was made an overview of Mixture Models. Then formalize the important EM algorithm, and clearly extend the discussion to a further formalization of the ZIP model integrated with the EM algorithm.
In Chapter 1, I introduce the mixture models, in particular describing the origin, interpretation and issues of this class of model. I finish the first chapter with interesting example about a mixture of two normal distribution with the real data of Old Faithful geyser. Chapter 2 is devoted to the formalization of basic EM Algorithm. I also finish this chapter with an example about transmission tomography. In Chapter 3, I present a brief introduction to ZIP model, in which I will travel through again themes already discussed in Chapter 1 and 2, but in this time referring to the ZIP model. I formalized the Maximum Likelihood Estimation using EM algorithm and I show Zero Inflated Poisson model fit in R. Finally in Chapter 4, I introduce my data and I do a descriptive analysis, with also a 3D plot and in the end I fit the ZIP model.
CONSULTA INTEGRALMENTE QUESTA TESI
La consultazione è esclusivamente in formato digitale .PDF
Acquista
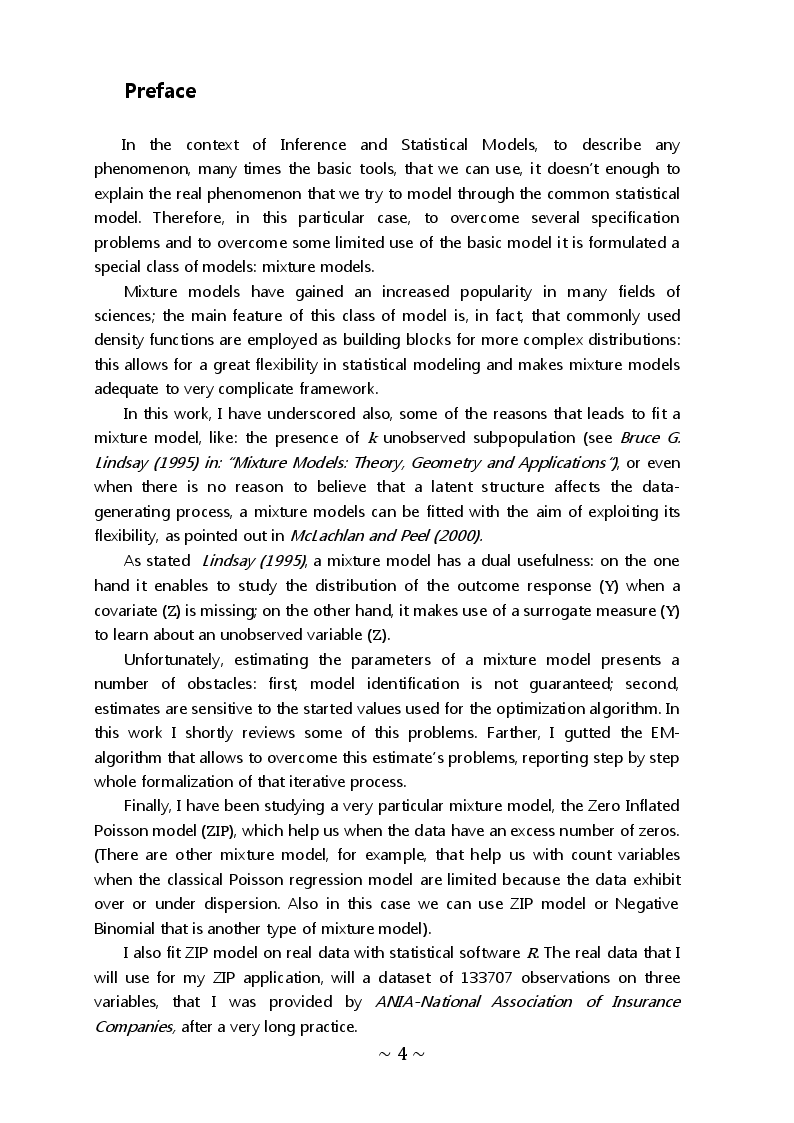
CONSULTA INTEGRALMENTE QUESTA TESI
La consultazione è esclusivamente in formato digitale .PDF
Acquista
Informazioni tesi
Autore: | Leonardo Affinito |
Tipo: | Laurea I ciclo (triennale) |
Anno: | 2012-13 |
Università: | Università degli Studi del Sannio |
Facoltà: | Scienze Statistiche ed Attuariali |
Corso: | L-41 |
Lingua: | Inglese |
Num. pagine: | 34 |
Forse potrebbe interessarti la tesi:
Tecniche statistiche algoritmiche per il monitoraggio del rischio di credito e l’individuazione dei fattori di rischio di default aziendale
FAQ
Come consultare una tesi
Il pagamento può essere effettuato tramite carta di credito/carta prepagata, PayPal, bonifico bancario.
Confermato il pagamento si potrà consultare i file esclusivamente in formato .PDF accedendo alla propria Home Personale. Si potrà quindi procedere a salvare o stampare il file.
Maggiori informazioni
Perché consultare una tesi?
- perché affronta un singolo argomento in modo sintetico e specifico come altri testi non fanno;
- perché è un lavoro originale che si basa su una ricerca bibliografica accurata;
- perché, a differenza di altri materiali che puoi reperire online, una tesi di laurea è stata verificata da un docente universitario e dalla commissione in sede d'esame. La nostra redazione inoltre controlla prima della pubblicazione la completezza dei materiali e, dal 2009, anche l'originalità della tesi attraverso il software antiplagio Compilatio.net.
Clausole di consultazione
- L'utilizzo della consultazione integrale della tesi da parte dell'Utente che ne acquista il diritto è da considerarsi esclusivamente privato.
- Nel caso in cui l’utente che consulta la tesi volesse citarne alcune parti, dovrà inserire correttamente la fonte, come si cita un qualsiasi altro testo di riferimento bibliografico.
- L'Utente è l'unico ed esclusivo responsabile del materiale di cui acquista il diritto alla consultazione. Si impegna a non divulgare a mezzo stampa, editoria in genere, televisione, radio, Internet e/o qualsiasi altro mezzo divulgativo esistente o che venisse inventato, il contenuto della tesi che consulta o stralci della medesima. Verrà perseguito legalmente nel caso di riproduzione totale e/o parziale su qualsiasi mezzo e/o su qualsiasi supporto, nel caso di divulgazione nonché nel caso di ricavo economico derivante dallo sfruttamento del diritto acquisito.
Vuoi tradurre questa tesi?
Per raggiungerlo, è fondamentale superare la barriera rappresentata dalla lingua. Ecco perché cerchiamo persone disponibili ad effettuare la traduzione delle tesi pubblicate nel nostro sito.
Per tradurre questa tesi clicca qui »
Scopri come funziona »
DUBBI? Contattaci
Contatta la redazione a
[email protected]
Parole chiave
Tesi correlate
Non hai trovato quello che cercavi?
Abbiamo più di 45.000 Tesi di Laurea: cerca nel nostro database
Oppure consulta la sezione dedicata ad appunti universitari selezionati e pubblicati dalla nostra redazione
Ottimizza la tua ricerca:
- individua con precisione le parole chiave specifiche della tua ricerca
- elimina i termini non significativi (aggettivi, articoli, avverbi...)
- se non hai risultati amplia la ricerca con termini via via più generici (ad esempio da "anziano oncologico" a "paziente oncologico")
- utilizza la ricerca avanzata
- utilizza gli operatori booleani (and, or, "")
Idee per la tesi?
Scopri le migliori tesi scelte da noi sugli argomenti recenti
Come si scrive una tesi di laurea?
A quale cattedra chiedere la tesi? Quale sarà il docente più disponibile? Quale l'argomento più interessante per me? ...e quale quello più interessante per il mondo del lavoro?
Scarica gratuitamente la nostra guida "Come si scrive una tesi di laurea" e iscriviti alla newsletter per ricevere consigli e materiale utile.
La tesi l'ho già scritta,
ora cosa ne faccio?
La tua tesi ti ha aiutato ad ottenere quel sudato titolo di studio, ma può darti molto di più: ti differenzia dai tuoi colleghi universitari, mostra i tuoi interessi ed è un lavoro di ricerca unico, che può essere utile anche ad altri.
Il nostro consiglio è di non sprecare tutto questo lavoro:
È ora di pubblicare la tesi