Software tools for the investigation of stochastic programming problems
Stochastic Programming (SP) has become synonymous with optimum decision making under uncertainty. However, modelling and solving stochastic programming problems are challenging tasks. Many factors affect the complexity of SP models with respect to their deterministic counterparts: (i) the need for capturing the random behaviour of the models parameters, (ii) the difficulties in solving the model instances (which are usually very large), and (iii) the requirements for advanced analysis of the model results through simulation techniques. This research focuses on the investigation of stochastic programming problems using software tools, and the outcomes of this work address some of the aforementioned difficulties. The well known classes of stochastic programming models and their properties are first reviewed, and a critical analysis of the use of algebraic modelling languages and their extensions for the formulation of SP models is presented. The insight gained into the interaction of models of randomness with optimisation models has led to the design of a novel approach to extending algebraic modelling languages for SP modelling. This approach has been successfully applied to the AMPL and MPL language to incorporate new SP language constructs. The resulting languages SAMPL and SMPL support the formulation of scenario-based SP problems in a natural and concise way. The integration of modelling systems with specialised solvers for SP has also been investigated. The use of decomposition methods for solving recourse problems has been analysed in terms of scale-up properties; alternative formulations of chance-constrained problems, which can be formulated as deterministic mixed integer programs, are also illustrated. Computational and conceptual issues related to the value of stochastic solutions (VSS) have been analysed and discussed. A Stochastic Programming Integrated Environment (SPInE), which supports the SMPL and SAMPL extended modelling languages and embodies a stochastic solver has been designed and developed in this research. The features of the system include the integration with scenario generators and the analysis of the SP model solutions and validation through the use of simulation. A case study is presented to illustrate how the SPInE system provides practitioners with a powerful platform for the analysis of real life problems using stochastic programming techniques.
CONSULTA INTEGRALMENTE QUESTA TESI
La consultazione è esclusivamente in formato digitale .PDF
Acquista
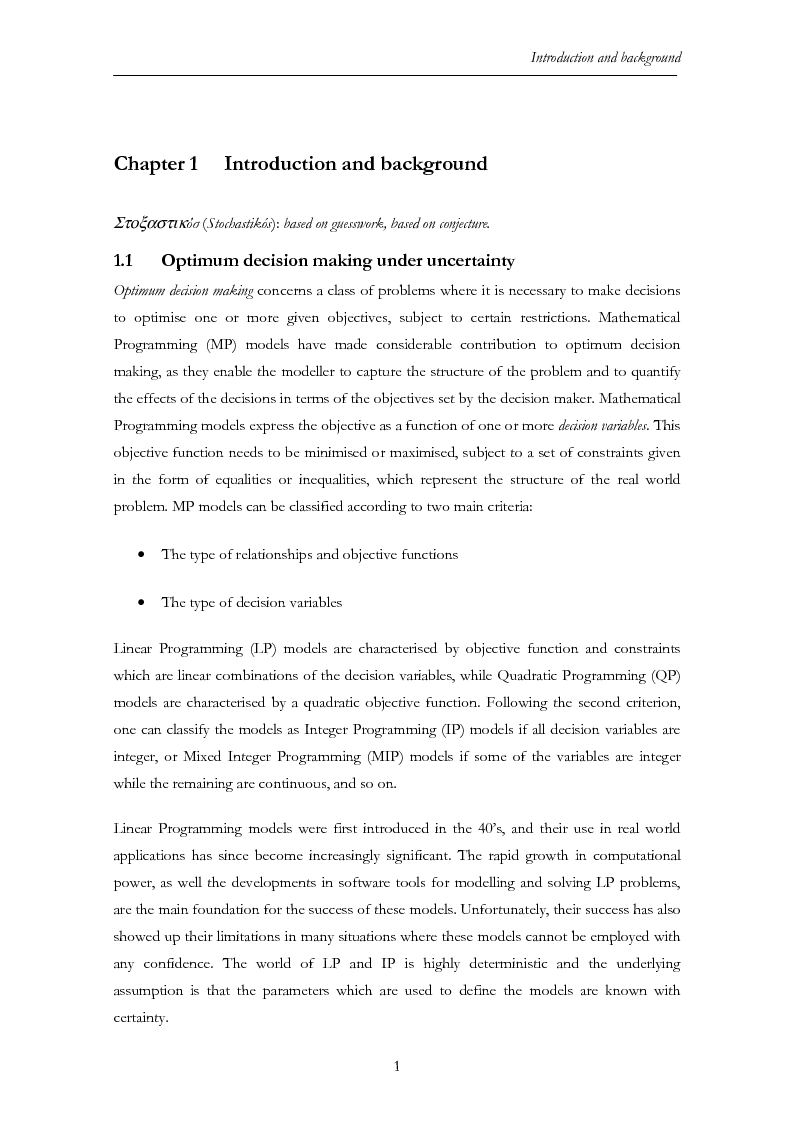
CONSULTA INTEGRALMENTE QUESTA TESI
La consultazione è esclusivamente in formato digitale .PDF
Acquista
Informazioni tesi
Autore: | Patrick Valente |
Tipo: | Tesi di Dottorato |
Dottorato in | Computational Modelling and Optimisation |
Anno: | 2002 |
Docente/Relatore: | Gautam Mitra |
Correlatore: | CormacLucas |
Istituito da: | Brunel University, London, UK |
Dipartimento: | Department of Mathematical Sciences |
Lingua: | Inglese |
Num. pagine: | 161 |
Forse potrebbe interessarti la tesi:
Mathematical programming applied to the decomposition of EMG signals
FAQ
Come consultare una tesi
Il pagamento può essere effettuato tramite carta di credito/carta prepagata, PayPal, bonifico bancario.
Confermato il pagamento si potrà consultare i file esclusivamente in formato .PDF accedendo alla propria Home Personale. Si potrà quindi procedere a salvare o stampare il file.
Maggiori informazioni
Perché consultare una tesi?
- perché affronta un singolo argomento in modo sintetico e specifico come altri testi non fanno;
- perché è un lavoro originale che si basa su una ricerca bibliografica accurata;
- perché, a differenza di altri materiali che puoi reperire online, una tesi di laurea è stata verificata da un docente universitario e dalla commissione in sede d'esame. La nostra redazione inoltre controlla prima della pubblicazione la completezza dei materiali e, dal 2009, anche l'originalità della tesi attraverso il software antiplagio Compilatio.net.
Clausole di consultazione
- L'utilizzo della consultazione integrale della tesi da parte dell'Utente che ne acquista il diritto è da considerarsi esclusivamente privato.
- Nel caso in cui l’utente che consulta la tesi volesse citarne alcune parti, dovrà inserire correttamente la fonte, come si cita un qualsiasi altro testo di riferimento bibliografico.
- L'Utente è l'unico ed esclusivo responsabile del materiale di cui acquista il diritto alla consultazione. Si impegna a non divulgare a mezzo stampa, editoria in genere, televisione, radio, Internet e/o qualsiasi altro mezzo divulgativo esistente o che venisse inventato, il contenuto della tesi che consulta o stralci della medesima. Verrà perseguito legalmente nel caso di riproduzione totale e/o parziale su qualsiasi mezzo e/o su qualsiasi supporto, nel caso di divulgazione nonché nel caso di ricavo economico derivante dallo sfruttamento del diritto acquisito.
Vuoi tradurre questa tesi?
Per raggiungerlo, è fondamentale superare la barriera rappresentata dalla lingua. Ecco perché cerchiamo persone disponibili ad effettuare la traduzione delle tesi pubblicate nel nostro sito.
Scopri come funziona »
DUBBI? Contattaci
Contatta la redazione a
[email protected]
Parole chiave
Tesi correlate
Non hai trovato quello che cercavi?
Abbiamo più di 45.000 Tesi di Laurea: cerca nel nostro database
Oppure consulta la sezione dedicata ad appunti universitari selezionati e pubblicati dalla nostra redazione
Ottimizza la tua ricerca:
- individua con precisione le parole chiave specifiche della tua ricerca
- elimina i termini non significativi (aggettivi, articoli, avverbi...)
- se non hai risultati amplia la ricerca con termini via via più generici (ad esempio da "anziano oncologico" a "paziente oncologico")
- utilizza la ricerca avanzata
- utilizza gli operatori booleani (and, or, "")
Idee per la tesi?
Scopri le migliori tesi scelte da noi sugli argomenti recenti
Come si scrive una tesi di laurea?
A quale cattedra chiedere la tesi? Quale sarà il docente più disponibile? Quale l'argomento più interessante per me? ...e quale quello più interessante per il mondo del lavoro?
Scarica gratuitamente la nostra guida "Come si scrive una tesi di laurea" e iscriviti alla newsletter per ricevere consigli e materiale utile.
La tesi l'ho già scritta,
ora cosa ne faccio?
La tua tesi ti ha aiutato ad ottenere quel sudato titolo di studio, ma può darti molto di più: ti differenzia dai tuoi colleghi universitari, mostra i tuoi interessi ed è un lavoro di ricerca unico, che può essere utile anche ad altri.
Il nostro consiglio è di non sprecare tutto questo lavoro:
È ora di pubblicare la tesi